CMU, DMI, and Module Design implement augmented reality to boost manufacturing line productivity
Nathan Snizaski
May 21, 2024
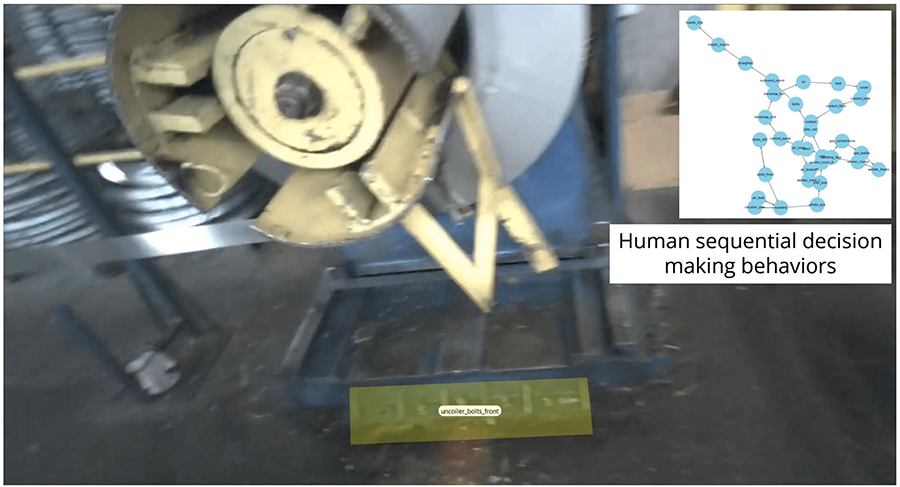
Automatic cognitive behavior decoding in real-time via Hololens
Pennsylvania-based industry partners DMI Companies (Charleroi, PA) and Module Design Inc. (Pittsburgh, PA) have been impacted by unexplained production breaks in manufacturing lines producing building modules and mechanical system elements, accounting for around 30 to 70% of the workdays. To tackle these production issues, DMI and Module Design partnered with Carnegie Mellon University (CMU) researchers to develop a scalable and adaptable approach to explain and reduce production breaks by observing the behaviors of top employees using machine learning and augmented reality (AR).
The research project centers on developing smart factories where machines and systems can communicate with employees in real time, enhancing manufacturing efficiency and productivity. Using sensors, machine learning, digital twins, and artificial intelligence, CMU and its industry partners, DMI and Module Design, aim to improve decision-making processes that reduce downtime, minimize waste, and boost output. This approach makes manufacturing more streamlined, adaptive, and responsive to dynamic conditions.
“The building construction industry is unique in catering to small orders with high customization,” says Dr. Pingbo Tang, associate professor of civil and environmental engineering at CMU. “To handle those smaller orders and customize the product, workers need better decision support tools to help them understand how to set up and monitor manufacturing machines and reduce waste to make the entire production system more flexible.”
In the manufacturing world over the past 20 years, there has been a shift to high mix/low volume, where individual units are designed with subtle differences from the previous unit. Even though the overall appearance of a given product appears similar, minor design differences require extra detail from workers to manufacture each unit or component properly.
Machine setup stoppages account for a large portion of production downtime. When a worker encounters a customized order, they need to set up the machine at their workstation to produce the item according to specifications, sometimes causing delays. The research team aimed to identify operational efficiencies by observing top workers and their behaviors using machine learning and translating that information into best practices.
“In our research, we’ve determined that an experienced worker can set up factory machines in a much shorter time with much less waste than less experienced workers and the current state of AI,” says Tang.
With this technology, now you can understand why a high-performing machine operator approaches their work and how that makes the entire process more efficient. When you get to see the convergence of human expertise and machine learning, we can clearly see its value in manufacturing.
Doug Gudenburr, COO, DMI Companies
Tang demonstrates his team’s work in a video of a machine setup from the perspective of an experienced worker on a factory floor. In the video, the worker sets up the machine based on specific product parameters. Using a Hololens headset, a computer vision algorithm automatically recognizes parts of the machine and superimposes different colored boxes with labels over specific parts using AR.
“The algorithm produces a network of attention transfer, where we can take a person's tacit knowledge, based on where their attention is directed, and convert it into more explicit explanations,” says Tang. The research team also recorded the audio of experienced workers talking through their thought process during the setup and studied it through an audio analysis.
“What’s interesting about our work is that it trains both workers and machines,” says Tang. The idea is to allow the computer to follow the worker’s process through the video and audio capture and explain why this person does the job well and how time and material waste is minimized. Then, the machine can reuse the knowledge and transfer it to another person through AR technology.
“It's exciting to see our best workers in action from their point of view,” says Doug Gudenburr, COO at DMI. “We’re watching them go through their routines as they set up the machine, and we can observe notable, efficient processes. From this, you can document that knowledge in your standard operating procedures (SOP) that you can give to other workers who have to step into a particular station or perhaps don’t have as much experience.”
Ankur Dobriyal, director of Off-Site Innovation at Module Design, says that implementing this technology in module home production would have significant benefits due to the sheer variety of items that go into building a house. From knobs and handles to sinks and wooden frames, a complete parts list for a given design can feasibly have an unlimited combination of components.
“If the model can recognize and pull up the right installation checklist, cut sheets, or any kind of information that will help the installer with the information that they need, that is another big win that can come out of this project,” says Dobriyal. “Especially in Modular construction, we have a lot of people who are cross-trained across different trades. Expecting our workers to know how to cut sheets and specific installation details on every single product is unrealistic. Being able to help them through machine learning and AR should help reduce material waste and boost productivity.”
We have to make it as easy as possible for our workers to do their jobs. We need to convey to them what to do and how to do it and point out exactly where they might be doing something incorrectly. Our manuals and our work instructions have to be very specific, and this project has helped us refresh our standard operating procedures.
Ankur Dobriyal, director of off-site innovation, Module Design Inc.
“This project has helped us tremendously by opening the eyes of our workers, the boots on the ground that are managing these machines,” says Gudenburr. DMI’s workers see this technology's potential to make their jobs easier and are excited when they get to work with it.
“This project will serve as a foundation for our long-term strategy,” says Gudenburr. “There's no question about incorporating these concepts from visualization and optimization in everything that we do to help us achieve smart manufacturing. We're attempting to apply what we’ve learned to all of our production lines and to our other facilities.”
“Even before we started implementing the technology, the workforce education component [of the project] has had the biggest impact,” says Dobriyal. He says that proving out productivity breaks with data captured by the research team has helped inform line workers of the need for process improvement, from additional training to relocating production materials.
“If you ask someone how much time they spend away from their stations to get materials or get help from a manager, they might estimate 10 to 20 percent,” says Dobriyal. “But 70% downtime displayed for us in a graph is a figure that gets their attention.”
Tang emphasizes that introducing machine learning and AR to DMI and Module Design will improve workers’ productivity, not replace them.
“Our research has demonstrated that experienced human workers perform better than machines,” says Tang. “The human trains the computer, and then the computer trains the new worker. You need both humans and machines working collaboratively in this process.”
By adding AR headsets to the factory floor, Tang believes that human workers can use their native intelligence more effectively, ultimately making the production system more flexible and scalable.